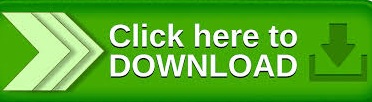
Statistical tests for stability and reliability were also conducted. COVLIAS 2.0 was validated using “Unseen NovMed” and benchmarked against MedSeg. Eight different pruning techniques (i) differential evolution (DE), (ii) genetic algorithm (GA), (iii) particle swarm optimization algorithm (PSO), and (iv) whale optimization algorithm (WO) in two deep learning frameworks (i) Fully connected network (FCN) and (ii) SegNet were designed. We hypothesize that by using pruning and evolutionary optimization algorithms, the size of the AI models can be reduced significantly, ensuring optimal performance. Ology: The proposed study uses multicenter ∼9,000 CT slices from two different nations, namely, CroMed from Croatia (80 patients, experimental data), and NovMed from Italy (72 patients, validation data). This study shows that COVLIAS 2.0 uses pruned AI (PAI) networks for improving both storage and speed, wiliest high performance on lung segmentation and lesion localization. It has issues related to storage space and speed. Further, we present a multi-ethnic, multi-center, racial bias-free study of strokeĬOVLIAS 1.0: an automated lung segmentation was designed for COVID-19 diagnosis.
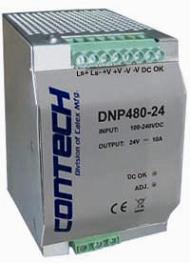
Model is beneficial in segmenting very bright and fuzzy plaque images that are hard to diagnose Similarly, the AUC value for Attention�UNet is 0.97, compared to 0.964, 0.966, and 0.965 for other models. The correlation coefficient (CC) value for Attention-UNet is 0.96, compared toĠ.93, 0.96, and 0.92 for UNet, UNet++, and UNet3P models. Visual plaque segmentation showed improvement in the Attention-UNet results compared to the Results of the Attention-UNet model are benchmarked against UNet, UNet++, and UNet3P models. The cross�validation K5 (80% training: 20% testing) protocol was applied for accuracy determination. Technique was applied to 679 CCA images, doubling the database for the experiments. WeĬombined both CCA images to form an integrated database of 679 images. Our experiments consist of 970 ICA images from the UK, 379 CCA images fromĭiabetic Japanese patients, and 300 CCA images from post-menopausal women from Hong Kong. That identifies the carotid plaques in the internal carotid artery (ICA) and common carotid artery This study proposes an attention-channel-based UNet deep learning (DL) model Artificial Intelligence-based methods ofĪutomatically detecting and predicting the severity of CVD and stroke in their early stages are of Methods are neither automated nor clinically accurate. TheĮarly detection of such events may prevent the burden of death and costly surgery. Stroke and cardiovascular diseases (CVD) significantly affect the world population.
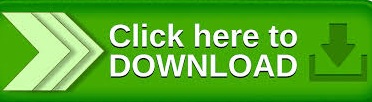